The Australian privacy act is changing – how could this affect your machine learning models?
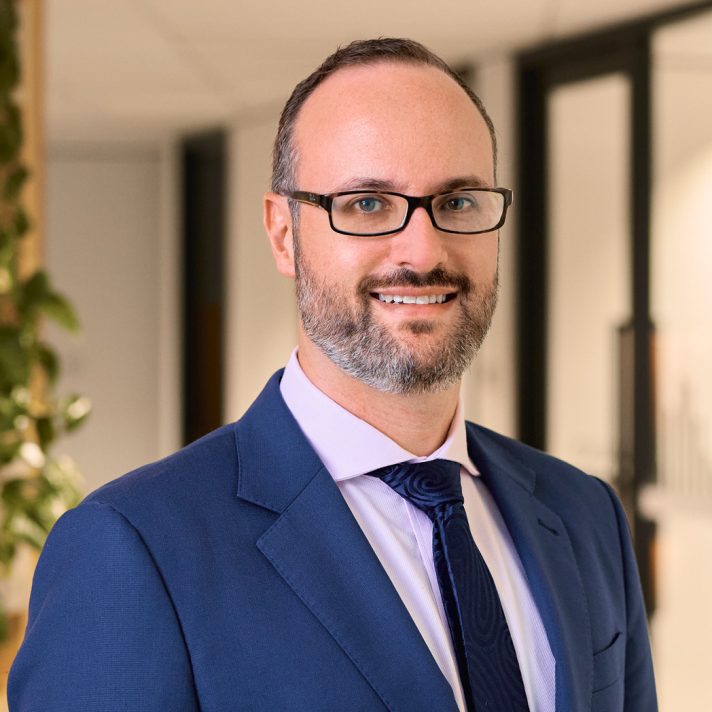
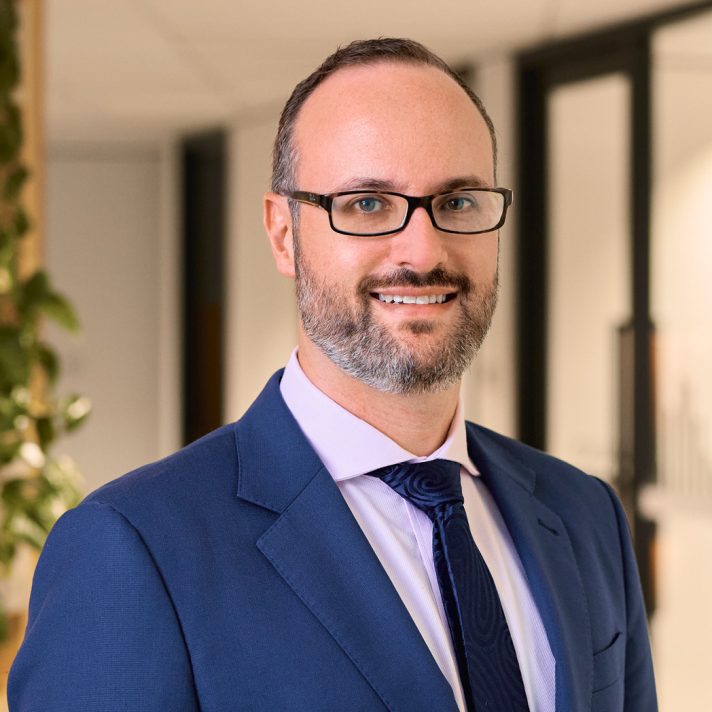
Countries across the globe are grappling with how to protect people’s privacy in the age of big data. In the first of a two-part series, we look at changes to the privacy act under consideration, and how to address the potential impacts for industry and consumers in a world increasingly tied to artificial intelligence and machine learning.
Community concerns have become more urgent in recent years regarding the way businesses collect, use and store people’s personal information. In response to a recommendation from the Australian Competition and Consumer Commission’s (ACCC) Digital Platforms Inquiry (DPI) – the Australian Government is reviewing the Privacy Act 1988 to ensure privacy settings empower consumers, protect their data and best serve the Australian economy.
Of the several proposed legislative changes, we outline three scenarios being considered and how they may impact organisations that collect and process customer data – in particular, those organisations that use machine learning algorithms in privacy-sensitive applications, such as predicting lifestyle choices, making medical diagnoses and facial recognition. We break down the three proposed changes to the privacy act below:
- Expansion of the definition of personal information to include technical data and ‘inferred’ data (information deduced from other sources)
- Introduction of a ‘right to erasure’ under which entities are required to erase the personal information of consumers at their request
- Strengthening of consent requirements through pro-consumer defaults, where entities are permitted to collect and use information only from consumers who have opted in.
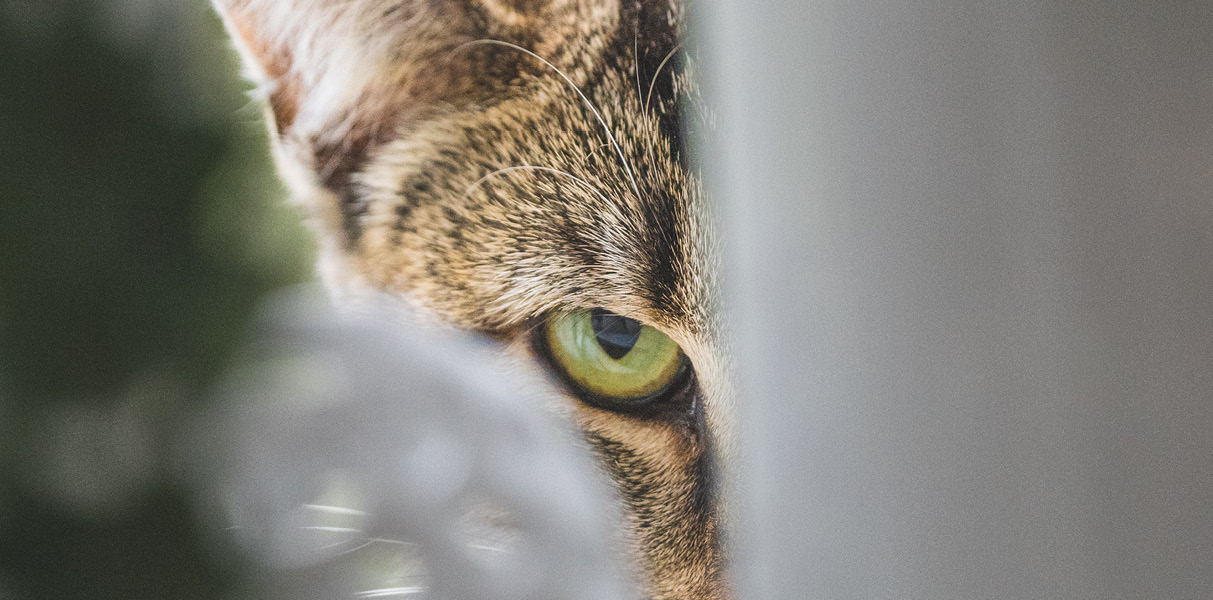
Algorithms used in privacy-sensitive applications such as lifestyle choices may be affected
1. Treating inferred data as personal information
As part of the proposed changes to the privacy act, the Government is considering expanding the definition of personal information to also provide protection for the following:
- Technical data, which are online identifiers that can be used to identify an individual such as IP addresses, device identifiers and location data.
- Inferred data, which is personal information revealed from the collection and collation of information from multiple sources. For example, a data analytics company may combine information collected about an individual’s activity on digital platforms, such as interactions and likes, with data from fitness trackers and other ‘smart’ devices to reveal information about an individual’s health or political affiliations.
How will this affect consumers, organisations and models?
The possibility of including inferred data as personal information in the privacy act represents a fundamental expansion in what organisations might think of as personal information. Typically, it is considered to be information provided by a user or trusted source that is known with a reasonable amount of certainty. For example, an organisation will know a customer’s age by requesting their date of birth.
In contrast, inferred information can usually only ever be known probabilistically. Under the proposed change, knowledge such as ‘there is an 80% probability that this customer is between 35 and 40’ could be treated the same way as knowledge such as ‘this customer is 37’.
This means model outputs may become ensnared in restrictive governance requirements because inferred customer information is often generated as an output of machine learning models. There is even a possibility that governance requirements may be extended to the models themselves, which have been shown to leak specific private information in the training data (data used to ‘train’ an algorithm or machine learning model) to a malicious attacker. The consequences for machine learning model regulation are potentially hugely significant for consumers and organisations, given personal information is subject to many more rights and obligations than the limited set currently applicable to models.
In addition, newly afforded rights for consumers may mean that they can request information on model origination or trading, and restrict future processing and use of models. On top of this, companies may now be required to design models that comply with data protection and security principles, and to discard models in order to comply with storage limitation principles.
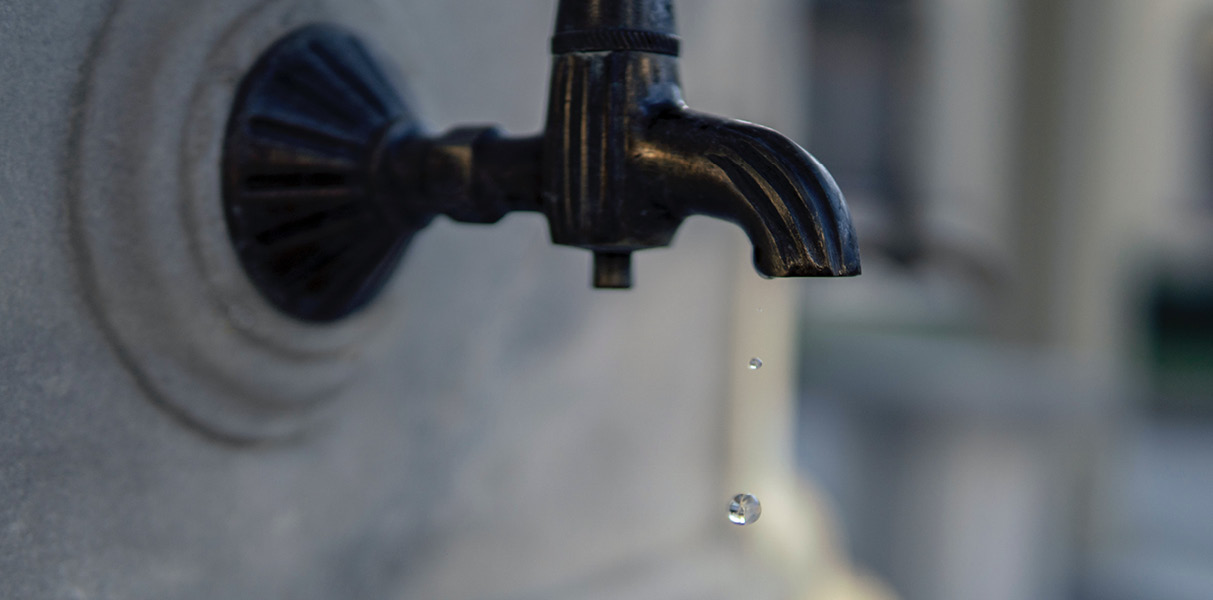
There are many challenges in protecting against unintended privacy leakages from models
When machine learning models leak private information
Privacy attacks on machine learning models can be undertaken with full access to a model’s structure (‘white box’ attack) or limited query access to a model’s observable behaviour (‘black box’ attack). Through this process, it is possible to infer probabilistic private information about an individual with a success rate significantly greater than a random guess.
Medical leaks
For example, US studies were performed on a regression model that predicted the dosage of Warfarin, a widely used anticoagulant, using patient demographic information, medical history and genetic markers. Researchers reverse engineered a patient’s genetic markers using only black-box access to the prediction model and demographic information about patients in the training data – with a success rate similar to a model trained specifically to predict the genetic marker.
Uncanny facial recognition
Recent studies have also demonstrated that recognisable images of people’s faces can be recovered from certain facial recognition models given only their name and black-box access to the underlying model to the point where skilled crowd workers could use the recovered photos to identify an individual from a line-up with 95% accuracy. The picture below on the left was recovered with only a name and limited ‘black box’ access to a model. It is eerily similar to the actual image on the right, which was used to train the model.
A problem with predictive text
Privacy leakage is particularly problematic for generative sequence models like those used for text auto-completion and predictive keyboards, as these models are often trained on sensitive or private data such as the text of private messages. For these models, the risks of privacy leakage are not restricted to sophisticated attacks, but may even occur through normal use of the model.
For example, model users may find that ‘my credit card number is …’ is auto-completed by the model with oddly specific details or even obvious secrets such as a valid-looking credit card number. Protecting against this sort of leakage is surprisingly challenging, with research indicating that unintended memorisation can occur early during training, and sophisticated approaches to prevent overfitting are ineffective in reducing the risk of privacy leakages.
Challenges in preventing privacy leakages
Machine learning models tend to memorise their training data, rather than learning generalisable patterns, unless specific care is taken in their construction – a phenomenon known as ‘overfitting’.
Unsurprisingly, theoretical and experimental results have confirmed that machine learning models, including regression and target classification models, become more vulnerable to privacy attacks the more they are overfit. What is a lot more surprising is that, while reducing overfitting can offer some protection, it does not completely protect against privacy leakage. Studies have found that models built using stable learning algorithms designed to prevent overfitting were also susceptible to leakage.
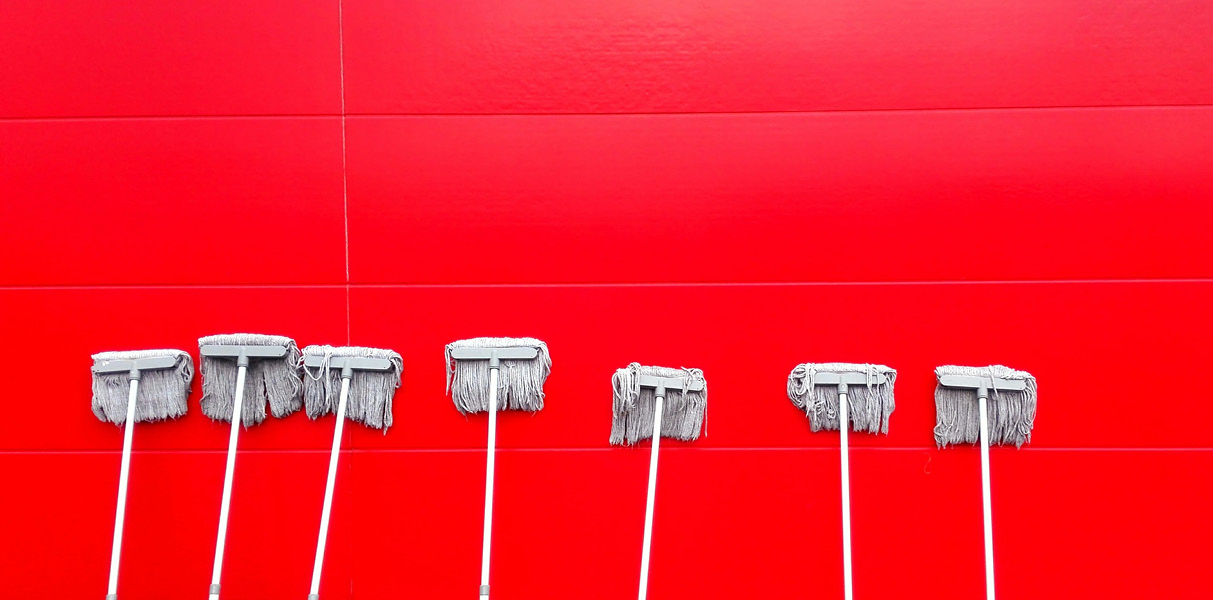
Consumers may gain the right to erase their data from a model, causing concerns for business
2. Right to ‘erasure’ – deleting personal information by customer request
Put into effect across the European Union in 2018 and sometimes known as the ‘right to be forgotten’, the broad ranging General Data Protection Regulations include a ‘right to erasure’, which provides European citizens with a right to have their personal data erased under certain circumstances, including when consumers have withdrawn their consent or where it is no longer necessary for the purpose for which it was originally collected and processed. This serves as a reference point for similar potential rights in the Australian Government’s review of the country’s privacy act.
Why organisations are cautious
Although organisations broadly support the introduction of a right to erasure, concerns have been raised about the potential negative impact the deletion of data may have on businesses and public interest. In its submission, Google considers it “important to include a flexible balancing mechanism or exceptions to such a right so as to enable businesses to consider deletion requests against legitimate business purposes for retaining data”.
Other organisations have proposed that any ‘right to erasure’ needs to be subject to appropriate constraints, which balance requests against legitimate business reasons, compliance with existing regulatory obligations and the technical feasibility and operational practicalities of implementation.
What could the ‘right to erasure’ mean for machine learning models?
Given machine learning models can be considered as having processed personal information, a consumer may wish to exercise their right to erase themselves from a model to remove unwanted insights about themselves or a group they identify as being part of, or to delete information that may be compromised in data breaches. Consumers may also seek to prevent the ongoing use of models that incorporate their data to prevent companies from continuing to derive a benefit from having historically held their information.
What would it mean for business?
In most circumstances, the removal of a single customer’s data is unlikely to have a material influence on a model’s structure. The ‘right to erasure’ becomes more powerful when exercised collectively through a co-ordinated action by a group of related customers, as their data is more likely to have had a material influence. For example, the use of facial recognition technology for identifying a marginalised group may cause a collection of customers to feel they are disadvantaged by the ongoing use of models trained on their data.
An immediate challenge is what constitutes a group before the right to erasure applies? Pragmatically, it would be difficult to define a general rule and it is plausible that even an erasure request from a single customer would require the removal of their data from the model, as well as their exclusion in the processing of data. This would be a very tall order for organisations to comply with, to the point of being unworkable in some situations where the cost and time required to comply with erasure requests rapidly outweighs the benefits of using a machine learning model at all.
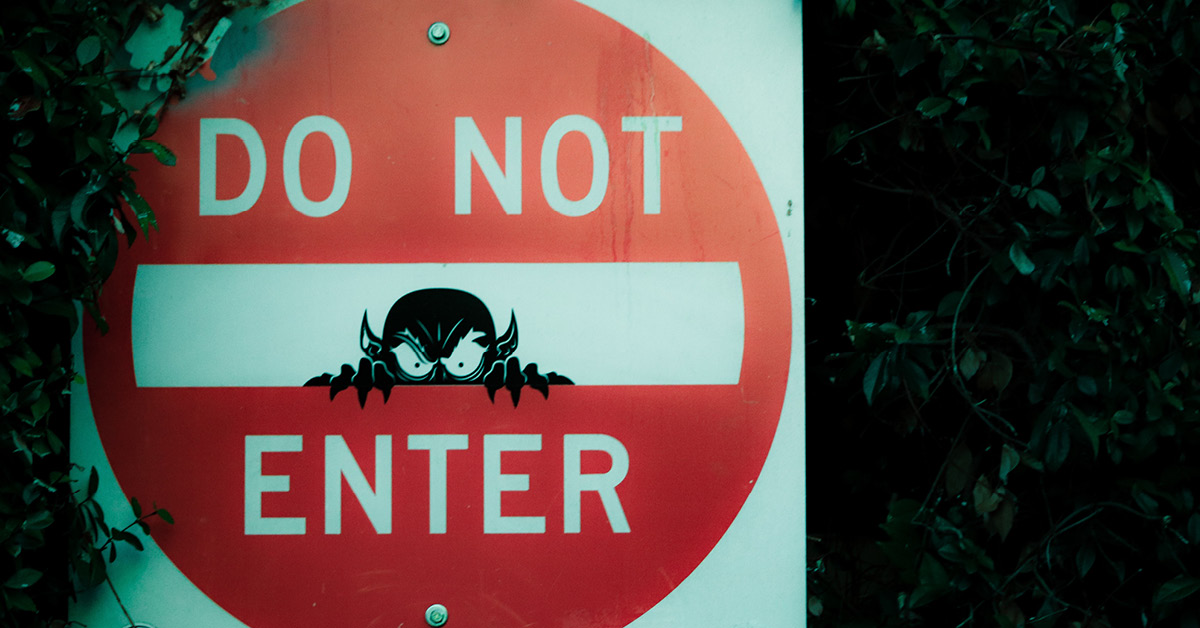
Organisations are cautious about proposals to pre-select digital consent settings to off
3. Strengthening consent – how will it affect model insights?
What pro-consumer defaults really mean
With consumers preferring digital platforms collect only the information they need to provide their products or services, the final ACCC Digital Platforms Inquiry report recommended that default settings enabling data processing for purposes other than contract performance should be pre-selected to off, otherwise known as ‘pro-consumer defaults’.
Why organisations are cautious
While organisations strongly support improved transparency for consumers through meaningful notice and consent requirements, they caution against proposals that would force businesses to provide long, complex notices or separate consents for each use of personal information. They argue that these approaches would cause consumers to switch off and not meaningfully engage with relevant privacy notices and controls, a phenomenon known as ‘consent fatigue’.
So do modellers have to start from scratch?
The potential implication of pro-consumer defaults is that the data available to organisations for future training of machine learning models may be limited, as it can be reasonably expected that relatively few consumers would make the effort to deliberately reverse these settings.
Consider an extreme scenario, whereby historical data that was collected prior to the introduction of the laws is no longer permitted to be used, through the new pro-consumer default settings. Many organisations may struggle in the weeks immediately following the change, and could effectively be required to rebuild models from scratch at a time when very little data may be available.
Could privacy protections lead to misleading model insights?
Problems could also arise where the profile and characteristics of customers that opt-in to data processing are materially different from the wider customer base who choose the default settings. These issues are likely to be more significant for analysis that is used to support operational and strategic decisions, as model insights may be based on a skewed view of customer behaviour.
Assessing your privacy risks – steps you can take now
With the review still underway, the final form of the changes made to the privacy act remain uncertain, with the potential ramifications for machine learning models even more so.
Nevertheless, there are some practical steps organisations can take now to assess and reduce potential privacy implications for their machine learning models and pipelines:
- Establish or review the composition of a cross-functional working group – The selection of key staff would define the technical, governance and compliance-related tasks that need to be completed, and identify who owns the process in areas such as analytics, privacy, legal, product owners and data.
- Review data usage policies – These should include explicit policies for how to use certain types of data and in which contexts, so that a consistent approach is maintained across the organisation.
- Track and document the use of customer data in models – This should include collating the following details for all existing models and pipelines: Original source of the data; applicable privacy statements for each data item and source; how and where the data is used in modelling processes; where possible, the contribution of each data source to model and organisational performance; if warranted, explicit testing of the impact on models of removing specific customer information, particularly for more sensitive information.
Taking these steps will provide organisations with a good view of where privacy risks may arise, and ensures they are better prepared for the potential implications of changes arising from the review of the privacy act.
In Part 2 of our ‘machine learning and the privacy act’ series, we explore the potential to apply existing data privacy approaches to machine learning models, what emerging approaches such as ‘machine unlearning’ have to offer, and the search for commercially robust solutions for complying with privacy requirements.
Other articles by
Jonathan Cohen
Other articles by Jonathan Cohen
More articles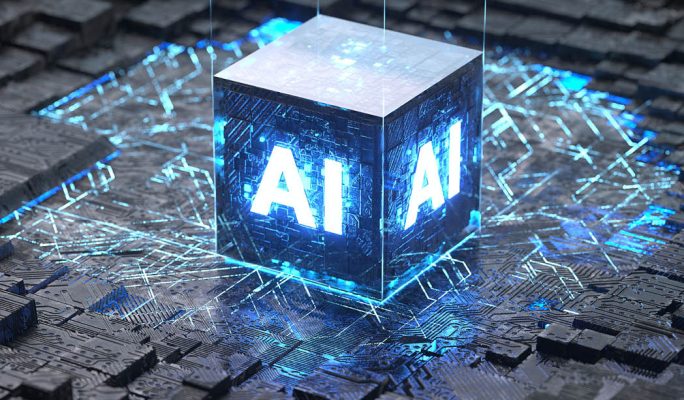
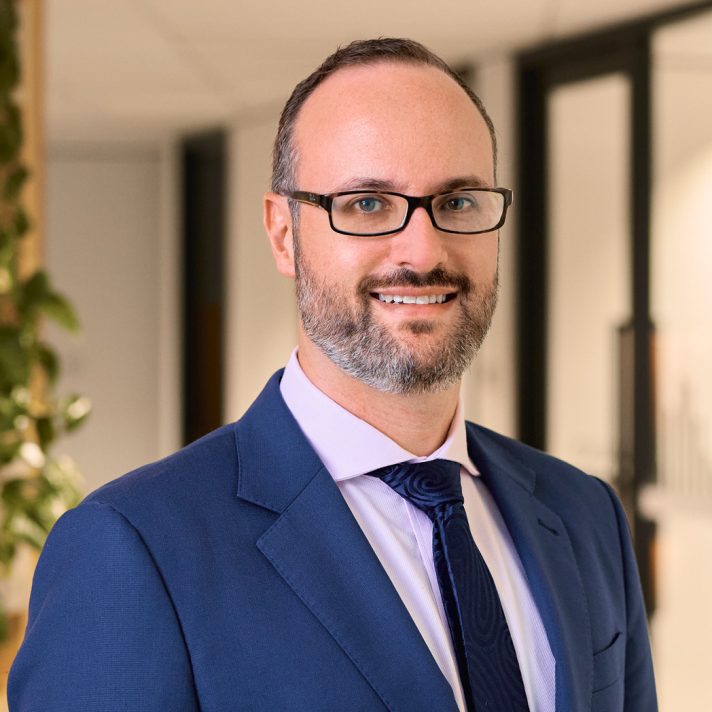
How AI is transforming insurance
We break down where AI is making a difference in insurance, all the biggest developments we’re seeing and what's next for insurers
Read Article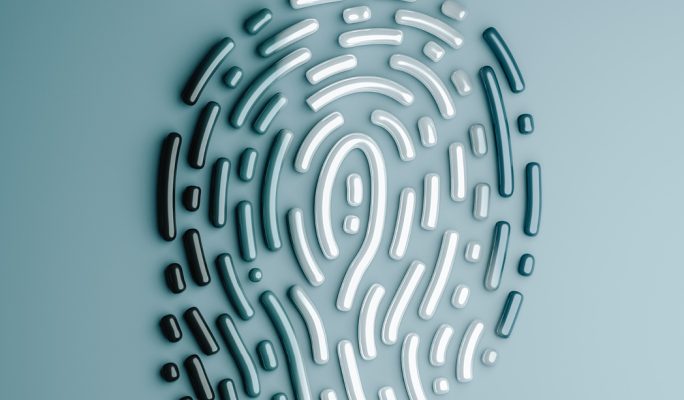
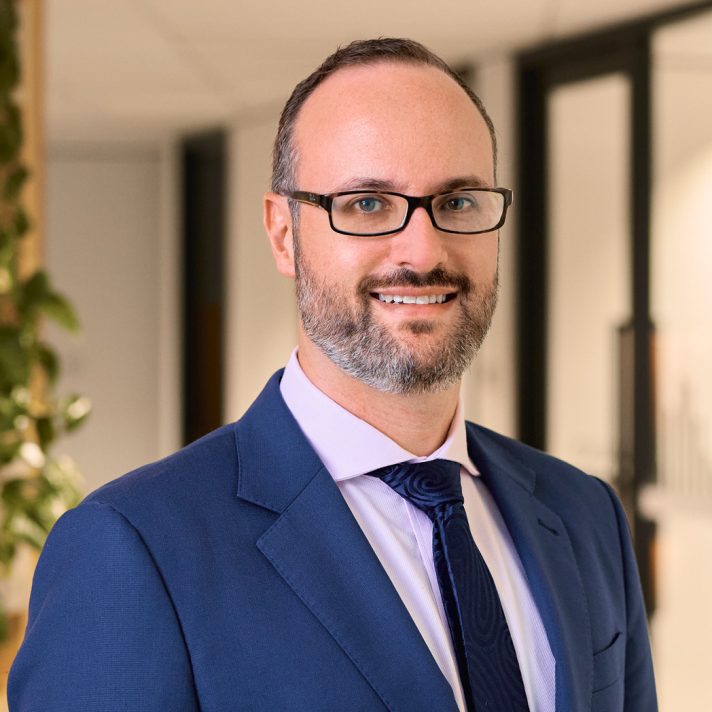
How AI will be impacted by the biggest overhaul of Australia’s privacy laws in decades
Understand the key changes to the Privacy Act 1988 that may impact AI and how organisations who use AI can prepare for these changes.
Read Article
Related articles
Related articles
More articles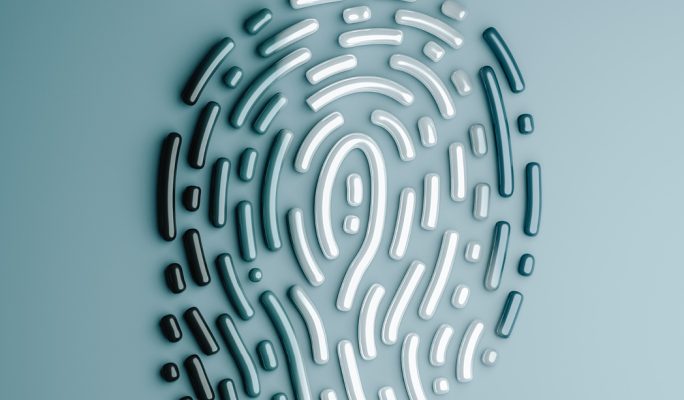
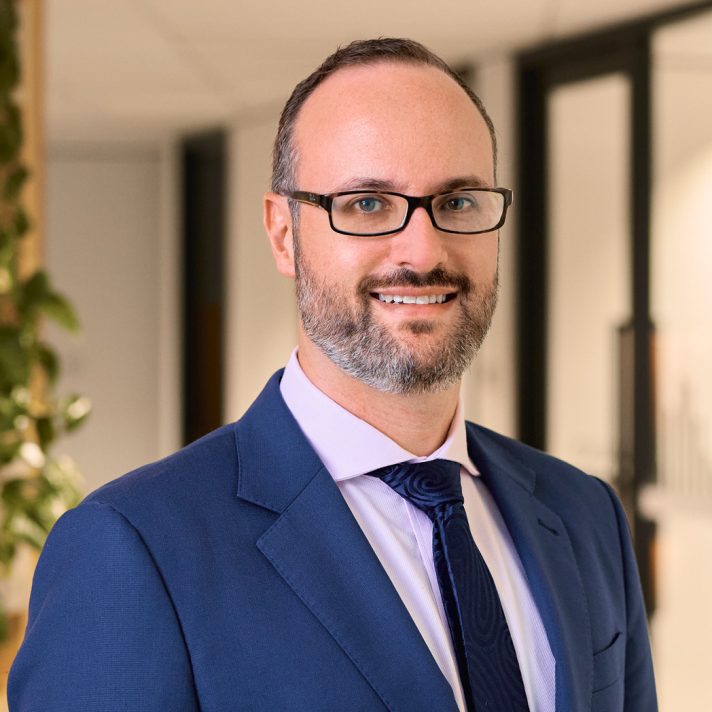
How AI will be impacted by the biggest overhaul of Australia’s privacy laws in decades
Understand the key changes to the Privacy Act 1988 that may impact AI and how organisations who use AI can prepare for these changes.
Read Article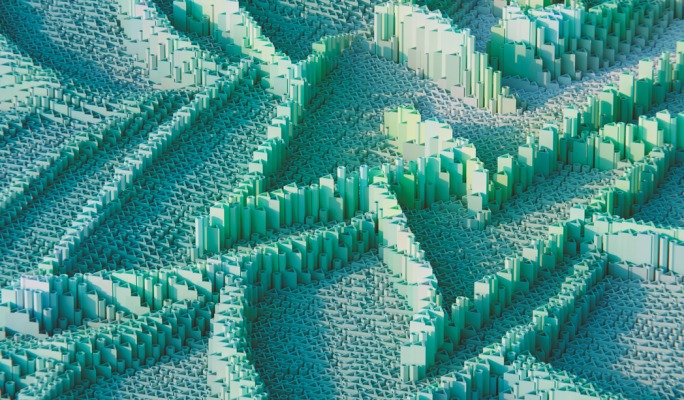
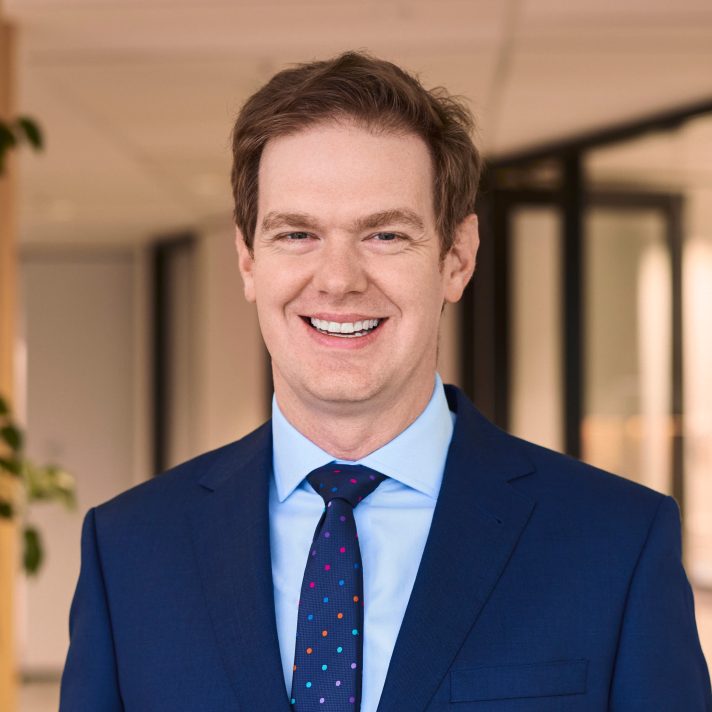
Well, that generative AI thing got real pretty quickly
Six months ago, the world seemed to stop and take notice of generative AI. Hugh Miller sorts through the hype and fears to find clarity.
Read Article